Machine Learning (ML) is attracting increased interest in credit risk modelling based on improved techniques, constantly growing computing power and, most recently, interest from regulators. Incorporating ML into Internal ratings-based (IRB) models is especially complex, incorporating numerous challenging aspects both in terms of an extensive regulatory landscape and the statistical properties of the models.
Against this background Advisense offers the “LGD Toolbox” which creates a set of thousands of possible IRB Loss Given Default (LGD) rank ordering models. Based on the models built it is possible to identify the best fit to compare and benchmark with an internal model. This is an efficient tool to find improvements to the internal model or determine that it has the right structure, the right risk drivers and therefore is the best predictor of risk.
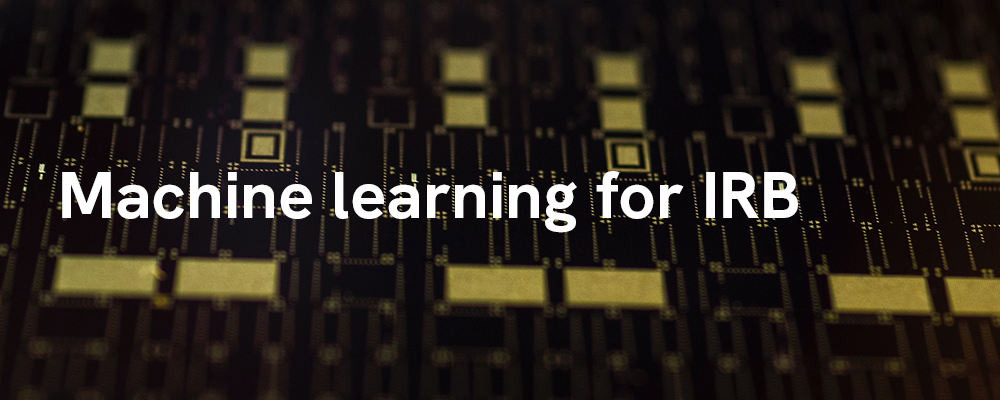
- Increased attention from regulators (e.g. EBA discussion paper on machine learning)
- The time is right to start making more informed decisions on the future use of ML in the organization’s risk modelling – we can help with that
- Benchmarking traditional models using ML is a strong comparison tool
- Possible future use in regulatory capital models, driven by the capital relief from superior risk models
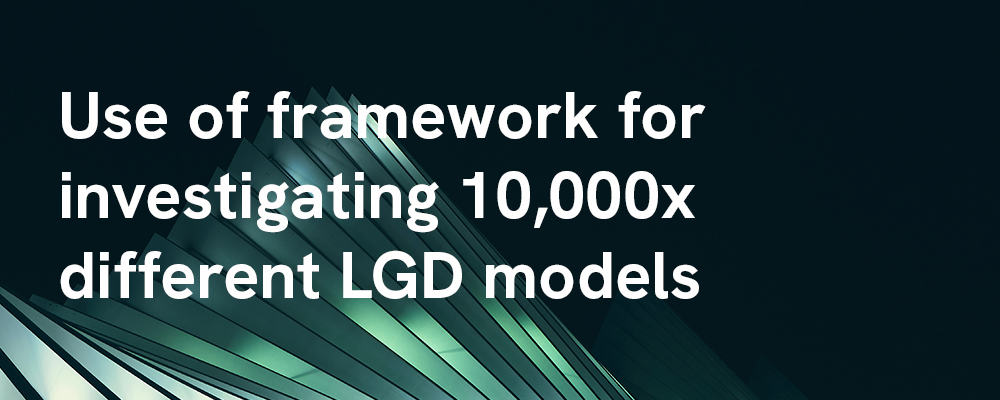
- The LGD Toolbox performs automated evaluation of 10,000+ different models
- Combination of different data transformations, model structures, ML techniques and risk drivers
- A flexible code structure enables an objective evaluation of the models in short amount of time
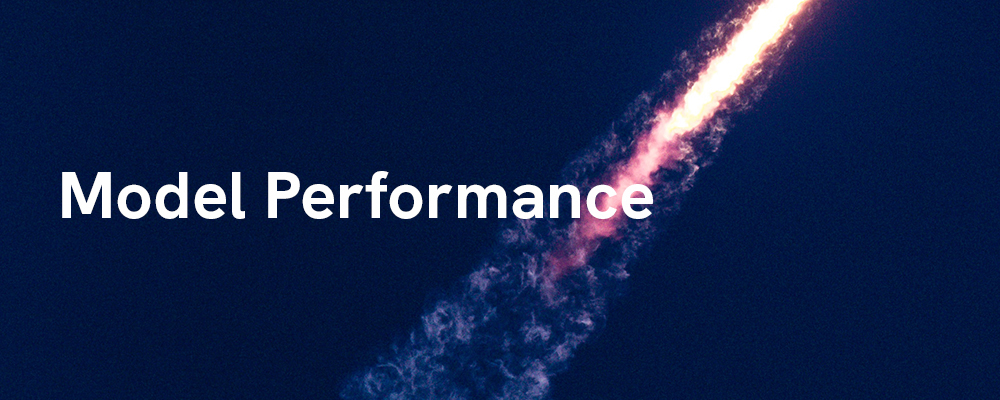
- The models built have a proven strong rank ordering ability
- The models built are able to capture the full LGD distribution by utilising a model structure based on different sub-models
- The measured performance of the best challenger model explains the maximum performance a bank can expect from an LGD model on that data set = near-theoretical performance ceiling
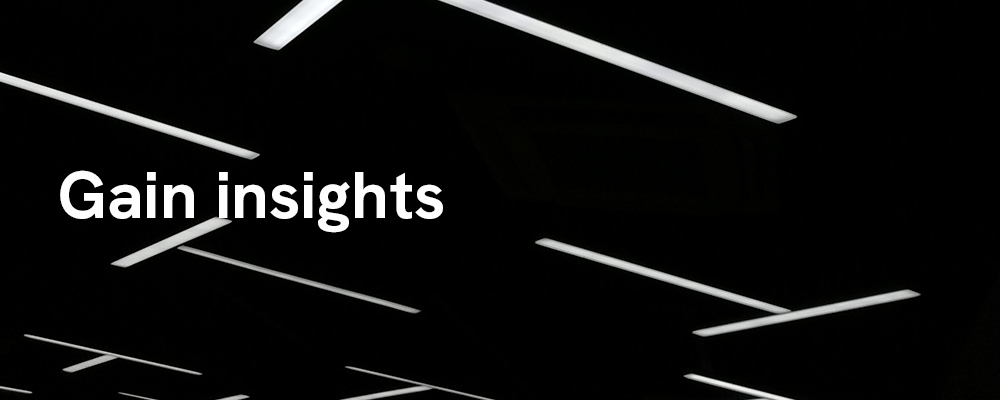
- By objectively searching all possible risk drivers in the data set, the LGD Toolbox provides a confirmation (or otherwise) of a bank’s LGD risk driver set
- A bank is able to consider improvements to its LGD models based on insights gained about the model structure (segmentation and use of sub-models)
- The LGD Toolbox utilises a wide range of ML techniques – understand what works best on your data and promote state-of-the-art technology for your modelling capabilities
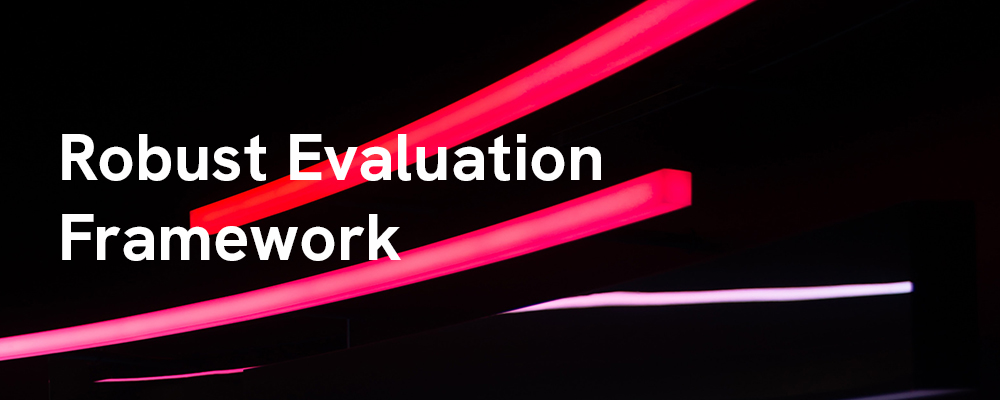
- The LGD Toolbox uses best practice comparison techniques and statistical measures
- The LGD Toolbox provides time stability analysis across multiple out of time samples or specific time periods within hold-out sample
- The performance evaluation is based on:
- Rank correlation (Somers’ D, Spearman)
- Error (MAE, RMSE)
- Classifier sub-models optimized on Brier score and also evaluated on AUC
- LGD prediction distribution (max-min diff)
- Test samples (Hold-out, Out-of-time)
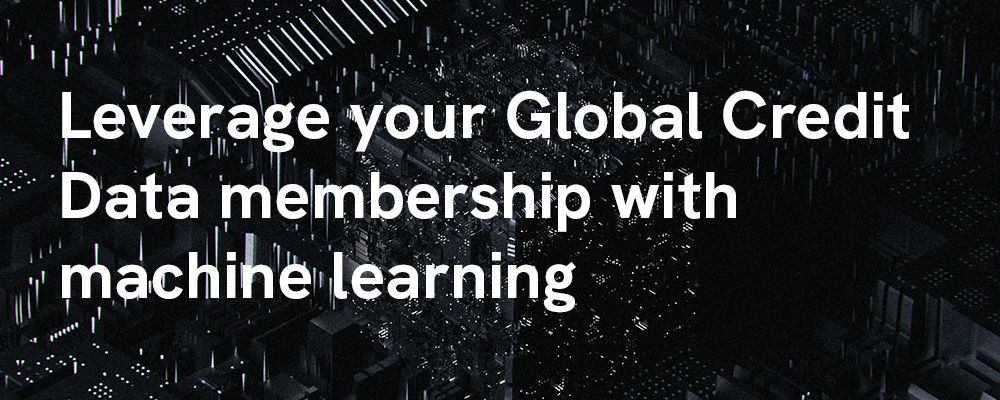
- Quick-start way to use GCD data (total data set or own bank subset)
- No installations, no integration, just deliver data – receive output
- For banks already having data in GCD’s standard format (best practice for data), this adds a companion “off the shelf” model which builds on GCD member discussion of best practice.
- Provides much deeper risk and modelling insights directly from anonymised internal data of member banks – an ideal “quick benchmark” tool for IRB banks, without the need of significant resources
Want to know more?
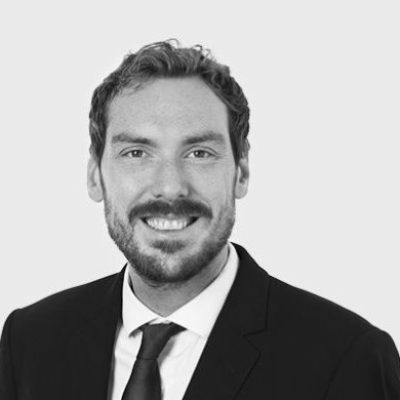
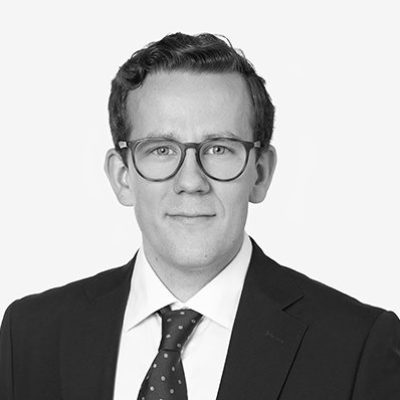