Anomaly Detection To Combat Fraud
Fraud accounts for at least 5–10 percent of all insurance claims payouts. Finding patterns in data that depart significantly from expected behaviour, to speed up fraud detection reduce costs is on top of the agenda in the non-life insurance sector. In this head-to-head game with fast-moving fraudster, companies are looking to strike the right balance between algorithms and human behaviour. Alexandra Baylis, data scientist and Senior Manager at Advisense, shares her thoughts on the way forward.
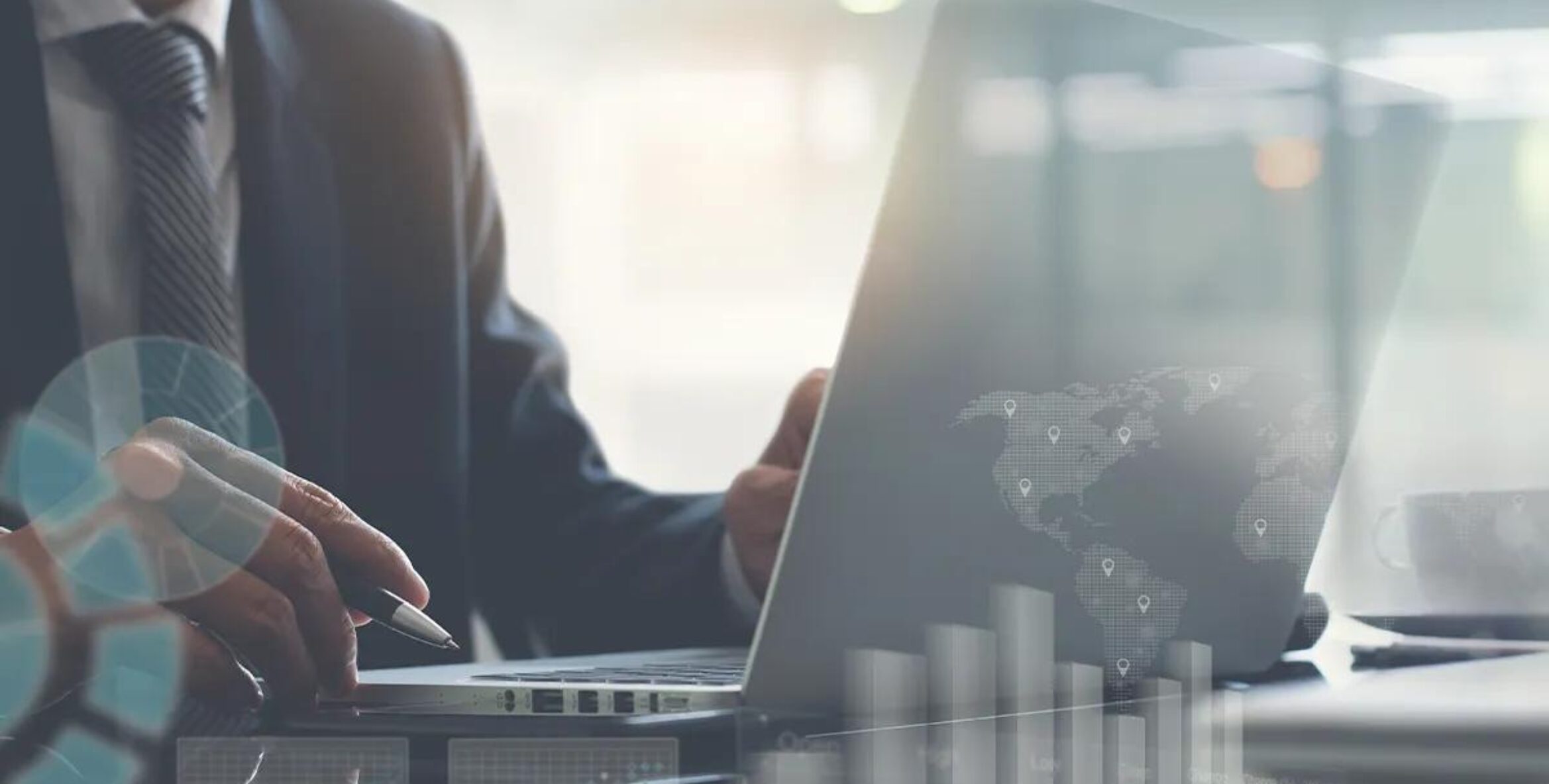
Spicing up the claims value for a broken camera, or systematic vehicle insurance fraud on through an insider? Every year, Swedish insurance companies handle more than three million claims and pay out approximately 70 billion SEK in compensation to their customers. A rough estimate is that insurance fraud accounts for at least 5–10 percent of these payouts, which amounts to roughly 3,5–7 billion SEK. The costs of fraud ultimately hit honest customers through increased premiums, and predictions are that fraud will continue to grow. It just might be that insurance companies who are best in class to counter fraud will be able to translate their efforts into a competitive edge, appreciated by both customers and shareholders.
Looking at research on insurance fraud some ten years ago, it is said that claims handlers can neither predict when someone will provide false information nor be certain when they are speaking with a policyholder attempting to commit fraud. A focus on historical claims data, means that the joint claims register (Gemensamt Skaderegister) is of central importance for the ability to detect certain types of fraudulent claims. In practice, the investigation process becomes directed towards individuals with a significant number of claims, “abnormal” behaviors (for example, belligerence and aggressiveness), and who report circumstances that appear either too unreasonable or too good to be true. Another practice from the past is to focus the investigation process on individuals who mismanage their premium payments.
The warning flags used by insurance companies (claims history, a focus on deviant behaviors, and the classification of customers into good and bad) can be dubious. According to earlier research, criteria are designed to detect similar cases, which in the end become elements of self-fulfilling prophecies.
But this is not where we are now, in 2025, right?
Hedging against fraud tactics with better models
The level of maturity and practices across the insurance industry varies. Insurance claims and claim reports are handled both automatic and manual. Some reports go directly through the system without manual review, while others require manual processing. Great technological progress has been made in recent years, which allows for a much better use of statistical models and their application in various environments. Existing rules-based models are being complemented and potentially later replaced with more advanced statistical models. Different types of models depending on the complexity of the task are being developed, to achieve a significant impact in terms of effective detection.
It is only logical that companies regardless of sector are more likely to dedicate time and resources into horizon scanning and trends analysis. Mapping out fraud risk within your business operations is critical and needs to be an ongoing endeavour to detect behaviour and tactics of fraudsters targeting specific products and services. Tactics shift continuously to exploit vulnerabilities and dodge detection. AI in the service of criminals is nothing new, and fraudsters are using increasingly sophisticated tools to select less obvious targets and slip through automated systems. In this head-to-head game, companies are poised to gear up with corresponding continuous refinement of models and updated algorithms based on new findings and historical data to identify new fraud patterns.
Optimally, algorithms should be activated every time something changes in a case, and automatic clearance for it to be moved forward in the system to ensure that the case has been reviewed and deemed free from fraud.
Feedback loops
According to Advisense experts, common challenges ten years ago are still there. I.e. managing the ins and outs of historical data and data quality. Depending on the availability of data and how good the data quality is, there can be some quite interesting ways to deal with this.
For example, if you only have a limited dataset, there are different methods to handle that and if your models do not find anything of relevance you can consider creating artificial data of the type that would have been interesting to find. Then, using that try to train your model to see if it generates any interesting results
Alexandra Baylis, Data Scientist and Senior Manager at Advisense
As insurance companies gain more experience – and this is happening fast – feedback loops should become more robust and efficient. Feedback loops are critical in the process of training models on all types of data, automated adjustments, followed by implementation and continuous monitoring of claims investigations.
It follows after implementation that fraud managers will want to closely monitor case investigations to understand how the models perform and identify areas that can be further improved based on new data and identified anomalies
Alexandra Baylis
To summarise, again, data quality is king. Adding the human factor, the fraud risk discourse needs to include the potential relationship between poor data quality and the risk of internal fraud, in other words willful manipulation of data in collusion with insurance fraudsters on the outside. In other words, can the use of manipulated data lead to a reduced possibility of detecting fraudulent practices through applied statistical models? Of course it depends on the volumes, however, to be clear, fraud, infiltration and corruption go hand in hand. On top of this, it needs to be recognized that employee safety can become an issue due to external attempts of manipulation and infiltration. More about internal fraud and corruption in our forthcoming article in this series.
Alexandra Baylis three pieces of advice going forward:
- Advanced statistical models are the future to tackle external fraud
- Data quality is key when using advanced statistical models
- Fraudsters are moving targets. Use feedback loops to continuously improve models
Learn more about our Financial Crime Prevention and Insurance services.
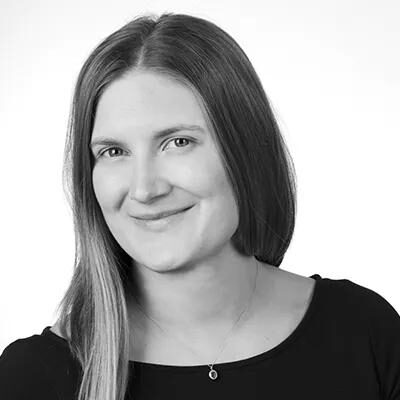
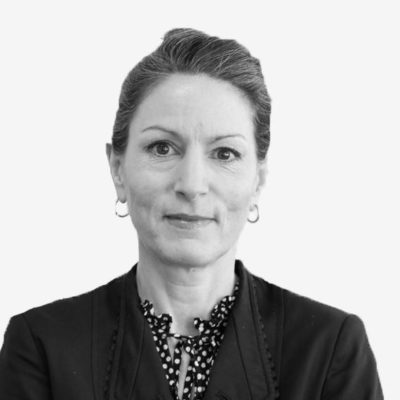