The three steps of cyber risk management improvement
What is the greatest risk, a medium or a medium?
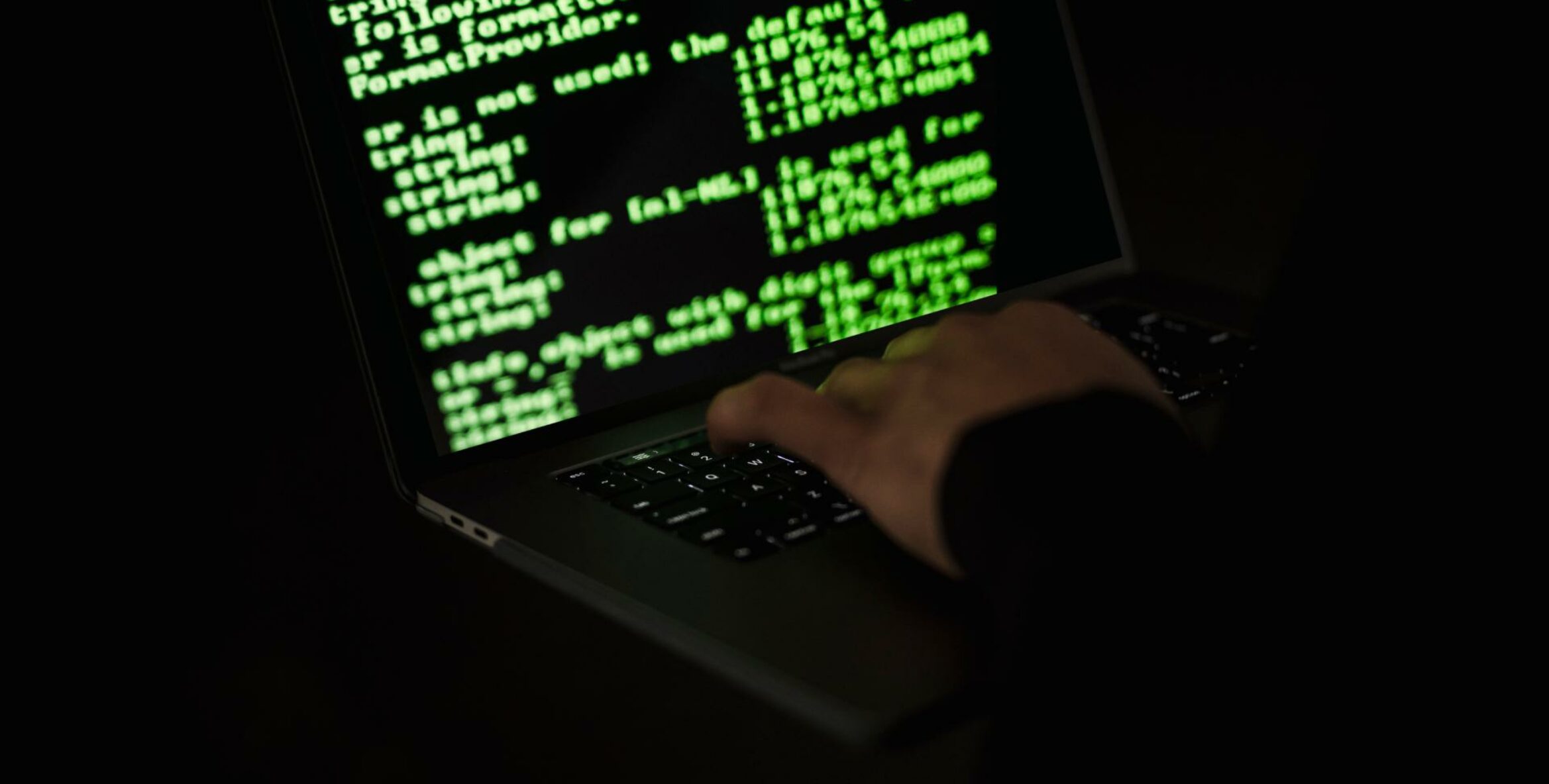
If you search for best practices in cyber risk management you almost always end up with the green, yellow and red heatmap. You assign a number to the probability and consequence and multiply them, and you get a number you can put somewhere on the heatmap. You understand that the risk with higher number is more important to put controls on but don’t really understand how important. So, you add percentage ranges for the probability and monetary ranges for the consequence. Now you understand better the risk to the business. After adding some risks, you end up with bunch of risks in the same square at the heatmap, so you increase the size of the heatmap to prioritize easier. You are happy because now you know your risks, and in which order you should put controls. Then you get three questions: What is our risk exposure? How much can we lose? If we spend 250 000 Swedish kronor, how much will the risk exposure decrease?
You answer that we have 50 medium and 7 high risks and if we spend 250 000 SEK we will end up with 45 medium and 5 high. But is that really an answer? Is 45 medium and 5 high in line with the risk acceptance? How much money is really at stake for the organization and with what probability?
A better answer would be: We have a probability of 5% of losing 1 000 000SEK or more within the next 12 months, this violates our risk acceptance. 250 000 SEK would get us down to 4% probability of losing 900 000SEK or more the next 12 months, which is in line with our risk acceptance.
I know what you are thinking, it’s impossible to know that. And you are right, it’s impossible to know that. But is 100% certainty needed? What you need is estimations you can trust, supports decision making and prioritization without ambiguous language. You can never know if 4% really is 4% as little as you can know a low is a low and not a medium. The biggest problem with the low is it can mean 1% probability to me and 15% to you, do we really see the risk in the same way then?
The heatmap has another limitation too. Every risk is managed separately, you can’t get a business wide visualization of the risk exposure. A simple but not perfect solution is described in the book “How to measure anything in cyber security risk” by Douglas W. Hubbard. Instead of assigning a score to the probability of a risk you set the probability in percent, for the consequence you assign a loss range you are 90% certain of (90% Confidence Interval, CI). You then add the risks to an excel sheet with monte carlo simulation. The result is presented as a loss exceedance curve (graph) expressing the risk exposure. Not perfect but a really good start in improving the cyber risk management.
The loss exceedance curve visualizes three things at the same time which makes it easy to understand, the inherent, the residual risk and the accepted risk. On an organization wide scale. This enables understanding of the total risk exposure without the ambiguous language of low, medium, high or a score. As the Confidence Interval (CI) express uncertainty, a security measure can be to reduce the uncertainty by collecting and analyze data, in other words, understand the risk better. The new knowledge might have a great effect on the risk exposure, not everything is hard security measures like new technology or routines.
For example, we have assigned a risk a CI of 100 000 – 20 000 000SEK. This express great uncertainty, we should probably assign resources to understand the risk better. After spending 20 hours in collecting and analyzing data we change the CI to 500 000 – 10 000 000SEK, this uncertainty reduction will have an effect on the loss exceedance curve and by that our risk exposure. So instead of directly trying put controls like creating a new routine or buy new equipment we affect the risk exposure just buy analyzing it more and reducing our uncertainty. Maybe the 20 hours spent saved us some money.
This method also enables minor estimation changes to the risk as we gain new knowledge. Move a risk from red to yellow on the heatmap usually require some major changes and feels like a daunting task. Changing a probability from 5% to 4,5% or CI from 100 000-400 000 to 120 000-380 000 require less effort. Small frequent changes is something Philip Tetlock in his research about forecasting found can increase the accuracy, he describes this in his book “Superforecasting”. So, maybe we can get better risk estimation accuracy by including risk assessment in our daily work instead of having one large yearly risk workshop.
This brings us to improving the estimations given by subject matters experts and others. Richards J. Heuers Jr. writes something worth a though in his book “Psychology of intelligence analysis”:
Analysis is, above all, a mental process. Traditionally, analysts at all levels devote little attention to improving how they think.
Richards J. Heuers Jr.
Philip Tetlock writes the following in “Superforecasting” about the learnings from his forecasting research project:
For it turns out that forecasting is not a “you have it or you don’t” talent. It is a skill that can be cultivated
Philip Tetlock
This is why a good start of the cyber risk assessment is to start with learning basic techniques in estimation and information analysis. Both the cyber risk framework FAIR and Hubbard advocate for calibration which from my experience works well. Calibration is a way to practice estimation techniques and improve your skills in estimating your own uncertainty. This means that, if you are good at estimating your own uncertainty and answer 10 question with 50% certainty you should have 5 correct answers (50%), if you are 80% certain you should have 8 correct answers. By measuring this we can understand how good the estimates given during the risk analysis are. We can never know if 4% probability really is 4% but we can know how good the person behind the number is at estimating. This brings us to a new learning from Philip Tetlock’s research: measurement is essential to improve estimates. In Tetlock’s research he found that experts are bad in forecasting and one reason may be that the accuracy of forecasts is never measured. He writes in his book Superforecasting:
“The consumers of forecasting — governments, business, and the public — don’t demand evidence of accuracy. So there is no measurement. Which means no revision. And without revision, there can be no improvement”
When the calibration is done, the risk workshop can be held but the workshop should only identify risks. The analysis should be performed individually for let’s say, two weeks. During these two weeks the persons giving estimates are encouraged to reduce his/her uncertainty by reading agreements, look at old incidents or infrastructure maps. After this they assign their probability and CI. How much they want to reduce their uncertainty is up to them as uncertainty reduction come at a cost (time is money). And the closer you get to absolute certainty the higher will the price be, the cost is exponential. Move from 50% certainty to 60% is cheaper than from 97% to 99%. But with continuous small changes to the risk you will continue improving estimation skills and reduce uncertainty.
To conclude, you can divide the cyber risk management improvement into three steps, of which the first two have been covered in this article. The first step is to start using a method which removes ambiguous language and enable organization wide aggregation and visualization of the total risk exposure. Step two is to avoid garbage-in, garbage-out by calibration, information analysis training and measurement. The more practice the better, but do not forget to measure to improve. As the last step a technical tool could help, the staff is trained in not putting garbage in, so the algorithms will not give garbage-out. But remember, analysis is above all a mental process.
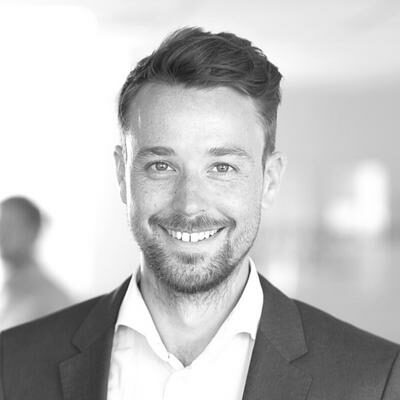